Advanced Diagnostics: Predictive Models to Detect Nutrient Deficiencies
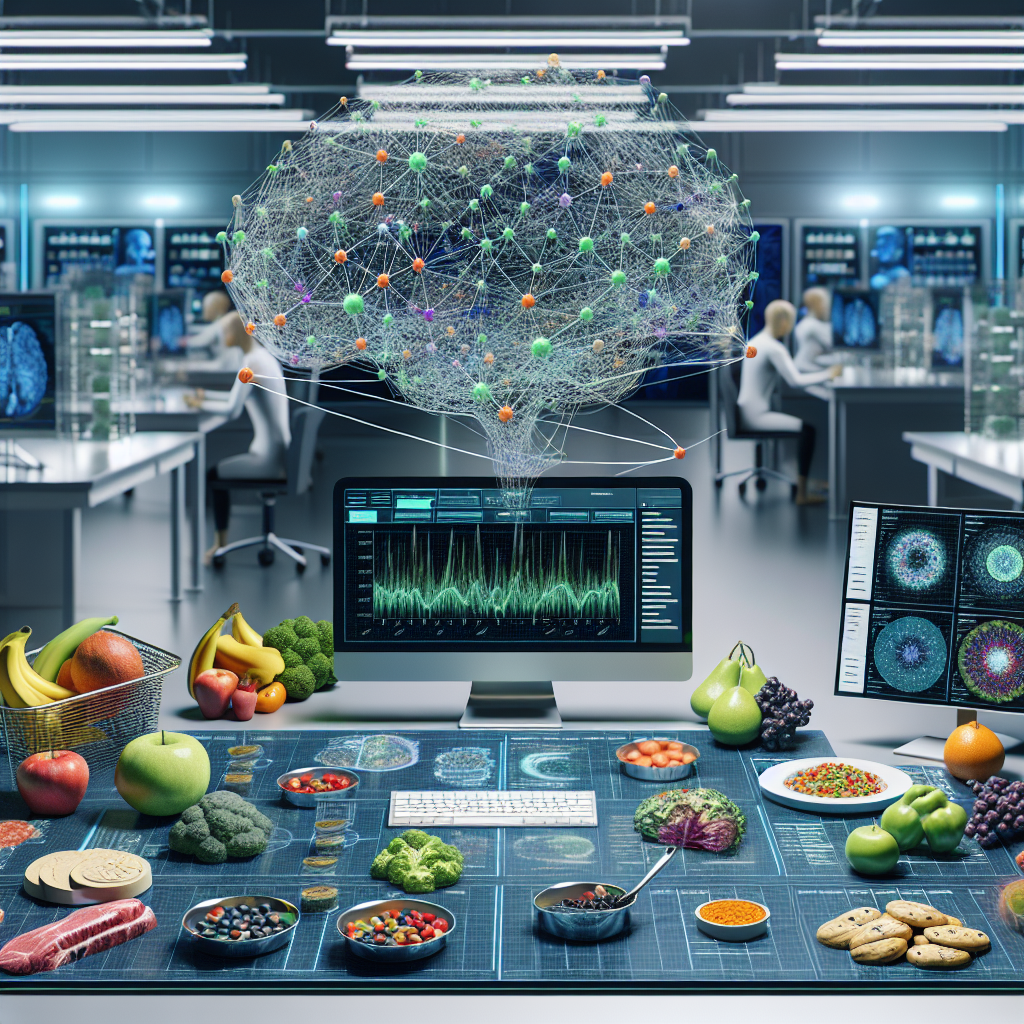
Unlock the power of advanced diagnostics with our cutting-edge predictive models designed to detect nutrient deficiencies before they impact your health. Take the first step towards a healthier you by visiting Vibrant Vitality today. Discover how our innovative approach can guide you to optimal wellness.
The Role of Machine Learning in Predicting Nutrient Deficiencies
In the realm of healthcare and nutrition, the advent of advanced diagnostics has marked a significant leap towards preemptive medical interventions. Among these advancements, predictive models, particularly those powered by machine learning (ML), have emerged as a cornerstone in detecting nutrient deficiencies, a common yet often overlooked condition that can have profound implications on an individual’s health. This article delves into the transformative role of machine learning in predicting nutrient deficiencies, shedding light on how this technology is reshaping our approach to dietary health and wellness.
Nutrient deficiencies, while preventable, remain a global health concern, affecting millions of people worldwide. Traditionally, the detection of these deficiencies relied heavily on physical symptoms and dietary assessments, methods that are not only subjective but also retrospective. However, with the integration of machine learning into the diagnostic process, the paradigm is shifting from reactive to proactive, enabling healthcare providers to identify potential deficiencies before they manifest into more severe health issues.
Machine learning, a subset of artificial intelligence (AI), excels in identifying patterns and making predictions based on large datasets. When applied to the field of nutrition, ML algorithms can analyze vast amounts of data, including dietary intake, genetic predispositions, and even lifestyle factors, to predict an individual’s risk of developing specific nutrient deficiencies. This predictive capability is not only more accurate but also significantly faster than traditional diagnostic methods, offering a promising avenue for early intervention and personalized nutrition plans.
One of the key strengths of machine learning in this context is its ability to process and learn from complex, multidimensional data. For instance, by analyzing blood test results alongside dietary records and physical activity logs, ML algorithms can discern subtle patterns that may indicate a risk of deficiency in certain vitamins or minerals. This holistic approach to data analysis allows for a more nuanced understanding of an individual’s nutritional status, beyond what can be gleaned from a single source of information.
Moreover, machine learning models are continually improving, thanks to their inherent ability to learn and adapt over time. As more data becomes available, these models can refine their predictions, becoming increasingly precise in identifying individuals at risk of nutrient deficiencies. This continuous learning process not only enhances the accuracy of diagnostic predictions but also contributes to the development of more effective, evidence-based nutritional guidelines and interventions.
The implementation of machine learning in predicting nutrient deficiencies also holds significant implications for public health. By enabling early detection and personalized intervention strategies, ML-powered diagnostics can help reduce the prevalence of nutrient-related health issues, such as anemia, osteoporosis, and immune system disorders. Furthermore, these advancements can contribute to more efficient allocation of healthcare resources, focusing efforts on prevention and early treatment rather than managing more advanced stages of deficiency-related diseases.
In conclusion, the role of machine learning in predicting nutrient deficiencies represents a pivotal development in the field of advanced diagnostics. By harnessing the power of AI to analyze complex datasets, ML algorithms offer a more accurate, efficient, and personalized approach to detecting and managing nutrient deficiencies. As this technology continues to evolve, it promises to further revolutionize our understanding of nutritional health, paving the way for more proactive and preventive healthcare strategies. In this era of digital health innovation, machine learning stands out as a key player in the quest for optimal nutrition and wellness.
Advancements in Nutrient Deficiency Diagnostics: From Blood Tests to Predictive Analytics
Advanced Diagnostics: Predictive Models to Detect Nutrient Deficiencies
In the realm of healthcare and nutrition, the ability to accurately diagnose nutrient deficiencies has traditionally hinged on the analysis of blood samples. These tests, while effective, offer a snapshot of a patient’s nutritional status at a single point in time. However, the landscape of diagnostics is undergoing a transformative shift, thanks to the advent of advanced predictive models. These innovative approaches are not only refining our understanding of nutrient deficiencies but also revolutionizing the way we detect and address them.
Predictive models in the context of nutrient deficiencies leverage a combination of artificial intelligence (AI), machine learning algorithms, and vast datasets to forecast potential nutritional shortfalls before they manifest into clinical symptoms. This proactive approach marks a significant departure from conventional diagnostics, which typically respond to deficiencies after they have already impacted an individual’s health. By analyzing patterns and trends in data, predictive models can identify individuals at risk of nutrient deficiencies, enabling early intervention and personalized nutrition plans.
One of the most compelling aspects of these predictive models is their ability to integrate diverse data sources. Beyond just blood tests, these models consider factors such as dietary intake, lifestyle habits, genetic predispositions, and even environmental influences. This holistic view of an individual’s health and nutritional status allows for a more nuanced understanding of their unique needs and risks. For instance, by recognizing the interplay between genetics and nutrient absorption, predictive models can identify individuals who may require higher intakes of certain nutrients due to genetic variations affecting metabolism.
Moreover, the application of machine learning algorithms in predictive diagnostics is a game-changer. These algorithms can sift through vast amounts of data, learning and adapting as they go, to uncover subtle patterns that may elude human analysts. For example, they might detect that individuals with specific dietary patterns and physical activity levels are more prone to certain deficiencies. This level of insight is invaluable for tailoring interventions and recommendations that are truly personalized, moving beyond one-size-fits-all dietary guidelines.
The transition from reactive to predictive diagnostics in nutrient deficiency detection also holds promise for public health initiatives. By identifying populations at risk of specific deficiencies, healthcare providers and policymakers can develop targeted nutritional programs and interventions. This could be particularly impactful in regions where certain deficiencies are prevalent, offering a strategic approach to nutritional health that is both preventive and cost-effective.
However, the shift towards predictive models is not without challenges. The accuracy of these models depends on the quality and breadth of the data they are trained on. There is also the need for ongoing validation and refinement of algorithms to ensure they remain relevant and reliable. Furthermore, ethical considerations around data privacy and the potential for bias in AI systems must be addressed to ensure these advancements benefit all segments of the population equitably.
In conclusion, the evolution of diagnostics from traditional blood tests to advanced predictive models represents a significant leap forward in our ability to detect and prevent nutrient deficiencies. By harnessing the power of AI and machine learning, these models offer a more proactive, personalized, and precise approach to nutritional health. As we continue to refine and expand these technologies, the potential for improving individual and public health outcomes is immense. The future of nutrient deficiency diagnostics is not just about detecting deficiencies; it’s about predicting and preventing them, marking a new era in healthcare and nutrition.
Integrating AI into Agriculture: Predictive Models for Early Detection of Plant Nutrient Deficiencies
In the realm of agriculture, the integration of advanced technologies has been a game-changer, particularly in the area of plant health and nutrient management. Among these innovations, the development and application of predictive models, powered by artificial intelligence (AI), stand out as a revolutionary approach to detecting nutrient deficiencies in plants. This advancement not only promises to enhance crop yield and quality but also contributes to sustainable farming practices by optimizing the use of fertilizers and reducing environmental impact.
The traditional methods of diagnosing nutrient deficiencies in plants often involve visual inspection and laboratory soil tests. While these approaches have their merits, they are not without limitations. Visual inspections can be subjective and may not accurately identify deficiencies until they have already caused significant damage to the plant. Soil tests, on the other hand, provide valuable information but can be time-consuming and do not offer real-time data. This is where AI-driven predictive models come into play, offering a more precise, efficient, and proactive approach to nutrient management.
Predictive models in agriculture utilize machine learning algorithms to analyze vast amounts of data from various sources, including satellite imagery, soil sensors, and weather data. By processing this information, these models can identify patterns and correlations that may not be immediately apparent to the human eye or through traditional analysis methods. For instance, a predictive model can analyze the color and shape of leaves, along with other environmental factors, to detect the early signs of nutrient deficiencies. This early detection is crucial as it allows farmers to address the issue before the plant’s health and yield are significantly compromised.
Moreover, these AI-driven models are capable of learning and improving over time. As more data is collected and analyzed, the models refine their predictions, becoming more accurate and reliable. This aspect of machine learning is particularly beneficial in the dynamic environment of agriculture, where conditions can change rapidly, and what worked one season may not be as effective the next.
The implementation of predictive models for detecting nutrient deficiencies also aligns with the principles of precision agriculture. By providing specific information about where and what type of nutrient deficiency is occurring, farmers can apply the exact amount of needed nutrients in targeted areas, rather than applying a uniform amount of fertilizer across an entire field. This not only reduces the cost of inputs but also minimizes the risk of over-fertilization, which can lead to nutrient runoff and environmental pollution.
Furthermore, the integration of AI into agriculture supports the broader goal of sustainable farming practices. By optimizing nutrient management, predictive models help to ensure that crops receive the right nutrients at the right time, enhancing plant health and productivity. This, in turn, can lead to higher crop yields with lower inputs, reducing the environmental footprint of farming and contributing to food security.
In conclusion, the advent of AI-driven predictive models for detecting nutrient deficiencies represents a significant leap forward in agricultural technology. These models offer a more accurate, efficient, and sustainable approach to managing plant health, promising not only to improve crop yields and quality but also to support the global challenge of sustainable agriculture. As technology continues to evolve, the potential for AI in agriculture expands, paving the way for a future where farming is more productive, environmentally friendly, and resilient.