Integrating AI in Precision Health: The Future of Diagnostics
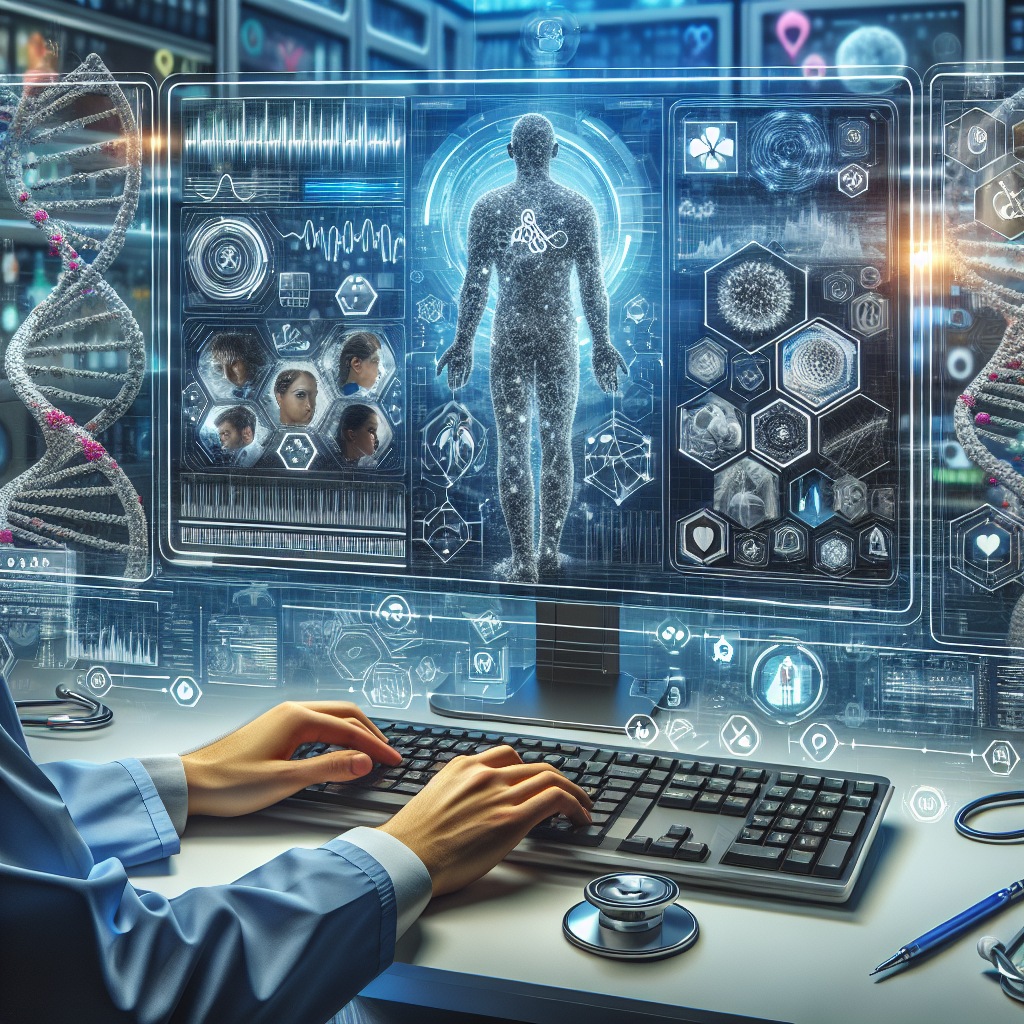
Discover the transformative power of integrating AI in precision health for the future of diagnostics. Embrace the cutting-edge advancements today and take a significant step towards personalized healthcare solutions. Learn more about how you can be part of this revolution.
Leveraging AI for Enhanced Diagnostic Accuracy in Precision Health
Integrating artificial intelligence (AI) into the realm of precision health marks a transformative leap towards enhancing diagnostic accuracy, heralding a new era in medical science. This integration not only promises to refine the precision with which health conditions are diagnosed but also to tailor treatments to the individual needs of patients, thereby optimizing outcomes. As we delve into the nuances of leveraging AI for enhanced diagnostic accuracy in precision health, it becomes evident that this technological advancement is not just an addition to the medical field but a revolutionary approach that could redefine patient care and treatment methodologies.
The journey of integrating AI into precision health diagnostics begins with the understanding that every patient’s genetic makeup, lifestyle, and environmental exposures are unique. Traditional diagnostic methods, while effective to a certain extent, often adopt a one-size-fits-all approach that may not capture these nuances. AI, with its ability to analyze vast datasets and identify patterns that may not be immediately apparent to human clinicians, offers a solution to this challenge. By harnessing the power of machine learning algorithms, AI can sift through genetic information, medical histories, and even real-time health data collected through wearable technology, providing a comprehensive analysis that can pinpoint potential health issues with astonishing accuracy.
Moreover, AI’s predictive capabilities are a boon for early detection of diseases. For instance, AI algorithms can analyze imaging scans to detect anomalies that are indicative of diseases such as cancer at a much earlier stage than traditional methods. This early detection is crucial, as it can significantly increase the chances of successful treatment and, in some cases, may even prevent the disease from developing further. The precision of AI in these scenarios not only enhances diagnostic accuracy but also reduces the likelihood of false positives or negatives, thereby minimizing unnecessary anxiety or invasive procedures for patients.
Transitioning from diagnosis to treatment, AI’s role in precision health becomes even more pronounced. Once a diagnosis is established, AI can assist in crafting personalized treatment plans by analyzing how similar cases have responded to various treatments. This not only ensures that patients receive the most effective care tailored to their specific condition but also helps in avoiding the trial-and-error approach often associated with determining the right treatment strategy. Furthermore, AI can monitor patient responses to treatment in real-time, allowing for adjustments to be made swiftly if the desired outcomes are not being achieved.
The ethical considerations and challenges associated with integrating AI into precision health cannot be overlooked. Issues such as data privacy, consent, and the potential for algorithmic bias necessitate rigorous scrutiny and the development of robust frameworks to ensure that the benefits of AI are realized without compromising patient rights or exacerbating health disparities. As these challenges are addressed, the potential for AI to revolutionize diagnostics and treatment in precision health becomes increasingly tangible.
In conclusion, the integration of AI into precision health represents a significant stride towards enhancing diagnostic accuracy and personalizing patient care. By leveraging the vast capabilities of AI, from analyzing genetic data to predicting disease progression and tailoring treatment plans, the future of diagnostics is poised to become more precise, effective, and patient-centered. As we continue to navigate the complexities of this integration, the promise of AI in transforming the landscape of healthcare and improving patient outcomes is undeniably bright.
The Role of Machine Learning in Predictive Analytics for Personalized Medicine
Integrating AI in Precision Health: The Future of Diagnostics
The advent of artificial intelligence (AI) in the healthcare sector has ushered in an era of unprecedented advancements, particularly in the realm of precision health. This innovative approach to medicine leverages the power of AI, especially machine learning (ML), to tailor healthcare to individual needs, thereby enhancing the accuracy of diagnostics and the efficacy of treatments. The integration of AI in precision health is not just a futuristic concept but a transformative reality that is reshaping the landscape of medical diagnostics and predictive analytics for personalized medicine.
Machine learning, a subset of AI, plays a pivotal role in this transformation. By analyzing vast datasets, ML algorithms can identify patterns and correlations that might elude human researchers. This capability is particularly valuable in the context of predictive analytics for personalized medicine, where the goal is to forecast health outcomes and tailor interventions to individual patients. The application of ML in this domain is multifaceted, ranging from genetic sequencing to the analysis of electronic health records (EHRs), each offering insights that contribute to a more nuanced understanding of patient health.
One of the most compelling applications of ML in precision health is its ability to analyze genetic data. By sifting through the complex web of genetic information, ML algorithms can identify mutations and genetic markers that predispose individuals to certain diseases. This information is invaluable, not only for predicting health outcomes but also for developing personalized treatment plans that address the unique genetic makeup of each patient. Furthermore, the integration of ML with genomics paves the way for the development of targeted therapies, marking a significant leap forward in the treatment of genetic disorders and cancers.
Moreover, ML’s analysis of EHRs offers another avenue for enhancing predictive analytics in personalized medicine. EHRs contain a wealth of information, including patient histories, lab results, and imaging data, which, when analyzed collectively, can reveal early warning signs of disease. ML algorithms excel at detecting these subtle patterns, enabling healthcare providers to intervene proactively and prevent the progression of disease. This not only improves patient outcomes but also reduces the burden on healthcare systems by mitigating the need for costly treatments and hospitalizations.
The integration of AI in precision health also extends to diagnostic imaging, where ML algorithms are revolutionizing the interpretation of medical images. By training on vast datasets of imaging scans, these algorithms can detect anomalies with a level of precision that rivals, and in some cases surpasses, that of human radiologists. This capability is particularly beneficial for the early detection of diseases such as cancer, where timely diagnosis can significantly improve survival rates.
In conclusion, the role of machine learning in predictive analytics for personalized medicine is both transformative and multifaceted. By harnessing the power of AI, healthcare providers can unlock insights hidden within genetic data, EHRs, and diagnostic images, thereby enhancing the precision of diagnostics and the personalization of treatment plans. As AI technologies continue to evolve, their integration into precision health promises to further refine our understanding of disease and revolutionize the future of diagnostics. This is not just a step forward in healthcare technology; it is a leap towards a future where medicine is tailored to the unique genetic blueprint of each individual, offering hope for more effective treatments and ultimately, better health outcomes.
Innovations in AI-driven Biomarker Discovery for Early Disease Detection
Integrating artificial intelligence (AI) into the realm of precision health marks a transformative leap forward in how we approach diagnostics and treatment. This integration is not merely an enhancement of existing methodologies but a revolutionary shift towards more personalized, efficient, and predictive healthcare. Innovations in AI-driven biomarker discovery for early disease detection are at the forefront of this transformation, promising a future where diseases can be identified and treated long before they manifest into more serious conditions.
The journey of integrating AI into precision health begins with understanding the vast potential of AI technologies in analyzing complex biological data. Traditional methods of biomarker discovery are often time-consuming and may lack the sensitivity and specificity required for early disease detection. However, AI, with its ability to sift through and make sense of large datasets rapidly, offers a solution to these challenges. By leveraging machine learning algorithms, researchers can now identify patterns and anomalies in genetic, proteomic, and metabolic data that would be impossible for human analysts to discern. This capability not only accelerates the pace of biomarker discovery but also enhances the accuracy and reliability of diagnostics.
Transitioning from the potential of AI in data analysis, it’s crucial to consider how these innovations are being applied in the real world. One of the most promising applications is in the early detection of cancers. Traditional screening methods for cancers, such as mammography or colonoscopy, have undoubtedly saved countless lives. However, they often detect cancer at later stages and can sometimes lead to false positives or negatives. AI-driven biomarker discovery is changing this landscape by identifying subtle, early signs of cancer in blood samples or imaging scans, long before tumors become visible or symptomatic. This early detection not only increases the chances of successful treatment but also significantly reduces the cost and invasiveness of interventions.
Moreover, the integration of AI in precision health extends beyond cancer to a wide range of diseases, including neurodegenerative diseases, cardiovascular conditions, and infectious diseases. For instance, AI algorithms are being developed to predict Alzheimer’s disease years before clinical symptoms appear, by detecting minute changes in brain imaging or subtle shifts in speech patterns. Similarly, in the realm of infectious diseases, AI-driven models are being used to predict outbreaks and identify novel pathogens rapidly, showcasing the versatility and breadth of AI applications in healthcare.
However, the journey towards fully integrating AI in precision health is not without its challenges. Issues such as data privacy, ethical considerations, and the need for robust validation of AI models must be addressed to ensure that these innovations benefit all segments of the population equitably. Furthermore, the successful implementation of AI in healthcare requires a multidisciplinary approach, involving collaboration between data scientists, clinicians, and policymakers.
In conclusion, the future of diagnostics in precision health is being reshaped by innovations in AI-driven biomarker discovery. This transformative approach promises a new era of early disease detection, where treatments can be more targeted, less invasive, and significantly more effective. As we navigate the challenges and opportunities presented by AI in healthcare, the ultimate goal remains clear: to harness the power of AI to improve health outcomes and quality of life for people around the world. The integration of AI in precision health is not just an exciting possibility—it is the pathway to a healthier future for all.