Machine Learning Models for Customizing Supplement Doses
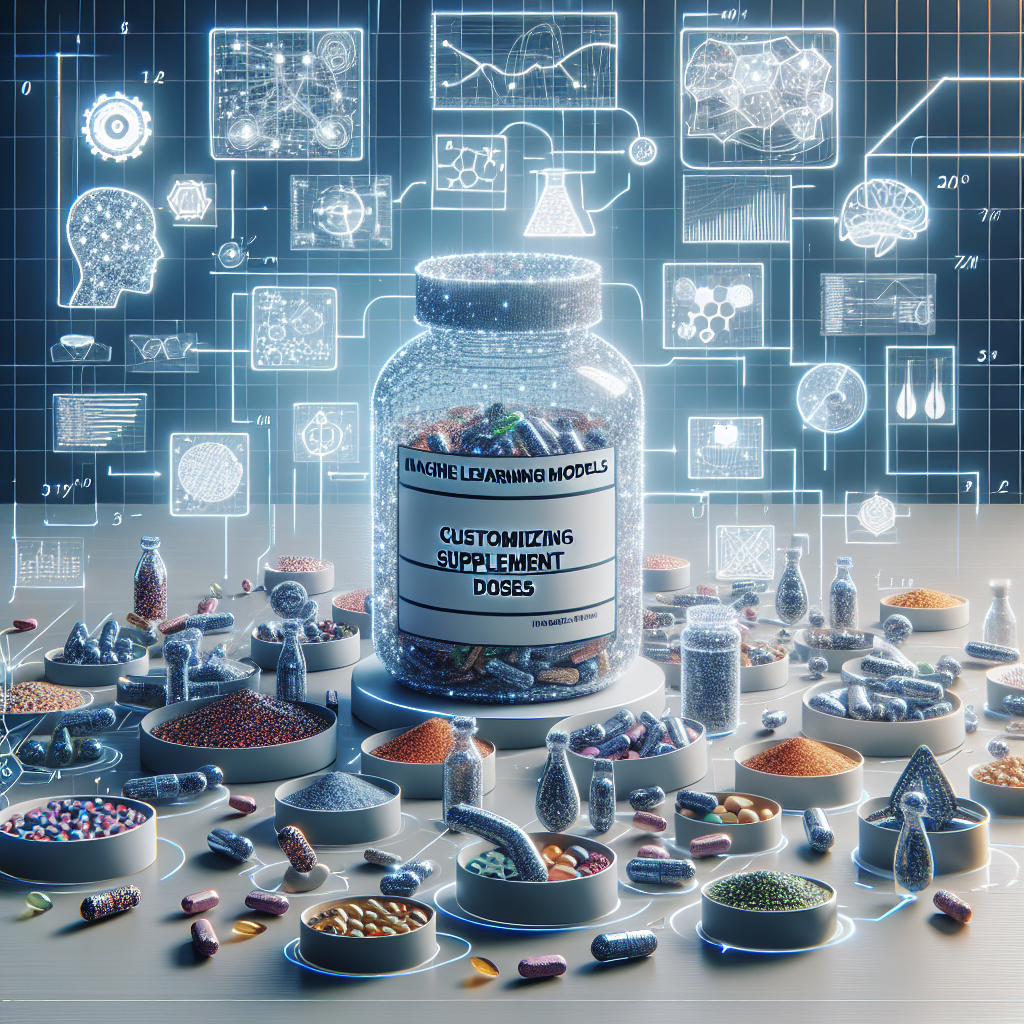
Unlock the full potential of your health with our cutting-edge Machine Learning Models, designed to customize your supplement doses for optimal wellness. Discover the future of personalized health now at Vibrant Vitality. Take the first step towards a healthier you today!
Optimizing Health: Leveraging Machine Learning Models for Personalized Supplement Dosages
In the realm of health optimization, the advent of machine learning models has ushered in a new era of personalized healthcare, particularly in the customization of supplement doses. This innovative approach is transforming how individuals manage their health, moving away from the one-size-fits-all mentality to a more tailored strategy that considers the unique physiological makeup of each person. By leveraging the power of machine learning, healthcare professionals and individuals alike can now fine-tune supplement regimens for optimal health outcomes.
The journey towards personalized supplement dosages begins with the collection and analysis of vast amounts of data. Machine learning models thrive on data; the more information these models can process, the more accurate and effective their predictions and recommendations become. In this context, data can range from genetic information and lifestyle factors to environmental influences and existing health conditions. By feeding this data into sophisticated algorithms, machine learning models can identify patterns and correlations that might not be immediately apparent to human analysts.
One of the most compelling aspects of using machine learning for customizing supplement doses is its dynamic adaptability. As new data becomes available, the models can update their recommendations in real-time, ensuring that individuals always receive the most current advice for their health needs. This is particularly beneficial in the context of supplements, where the body’s requirements can change due to factors like aging, stress, dietary changes, or the onset of new health conditions.
Moreover, machine learning models can account for the complex interactions between different supplements and how they might affect an individual’s health. For instance, certain combinations of vitamins and minerals can enhance absorption and efficacy, while others might inhibit it. Machine learning algorithms can analyze these interactions on a scale and with a precision that is simply not feasible for humans to achieve manually. This ensures that individuals are not only receiving the right supplements but are also taking them in combinations that maximize their benefits.
The potential of machine learning in customizing supplement doses also extends to predicting potential deficiencies before they manifest into health issues. By analyzing trends in the data, these models can forecast future nutritional needs and suggest adjustments to supplement dosages accordingly. This proactive approach can help prevent deficiencies and maintain optimal health, reducing the risk of related health problems down the line.
However, the implementation of machine learning models in customizing supplement doses is not without its challenges. Privacy concerns, data security, and the need for continuous updates to the algorithms are significant considerations. Additionally, the effectiveness of these models depends heavily on the quality and quantity of the data they are trained on, highlighting the importance of comprehensive and accurate data collection.
In conclusion, the integration of machine learning models into the customization of supplement doses represents a significant leap forward in personalized healthcare. By harnessing the power of data and the precision of algorithms, individuals can optimize their health in ways that were previously unimaginable. As technology continues to evolve, the potential for even more refined and accurate personalization in healthcare is vast, promising a future where everyone can achieve their optimal health through tailored supplement strategies.
The Future of Nutrition: How Machine Learning is Revolutionizing Supplement Customization
Machine Learning Models for Customizing Supplement Doses
In the rapidly evolving landscape of nutrition science, the advent of machine learning is heralding a new era of personalized health and wellness. The traditional one-size-fits-all approach to dietary supplements is being challenged by innovative technologies that promise a future where supplement doses are tailored to the individual needs of each person. This transformation is not just a leap forward in how we think about nutrition; it’s a paradigm shift that places the unique biochemical makeup of the individual at the center of dietary planning.
Machine learning models, with their ability to sift through vast datasets and identify patterns that elude human analysts, are at the forefront of this revolution. These models can analyze data from a variety of sources, including genetic information, lifestyle factors, and real-time health indicators, to predict the optimal mix and dosage of supplements for each individual. This level of customization is unprecedented and has the potential to significantly enhance the efficacy of dietary supplements.
The process begins with the collection of data. By leveraging wearable technology and other health monitoring devices, machine learning algorithms can access a continuous stream of health data from individuals. This data, when combined with genetic information and dietary habits, provides a comprehensive picture of an individual’s health status and nutritional needs. Machine learning models then use this data to identify correlations and patterns that can inform personalized supplement regimens.
One of the key advantages of this approach is its dynamic nature. Unlike static dietary recommendations, machine learning models can adjust supplement doses in real-time based on changes in an individual’s health data. This means that as a person’s health status, lifestyle, or environment changes, so too can their supplement regimen, ensuring that it remains optimally tailored to their current needs.
Moreover, machine learning models can also account for the synergistic and antagonistic relationships between different nutrients. This is a complex area of nutrition science that is difficult for humans to navigate due to the sheer number of possible interactions. Machine learning algorithms, however, can analyze these interactions at scale, ensuring that supplement regimens are not only personalized but also balanced and safe.
The potential benefits of this technology are vast. For individuals, it promises more effective and efficient nutritional support, potentially leading to better health outcomes and a higher quality of life. For healthcare providers, it offers a tool to deliver more personalized care, enhancing patient satisfaction and engagement. And for the supplement industry, it opens up new avenues for product development and differentiation in a crowded market.
However, the path to widespread adoption of machine learning in supplement customization is not without challenges. Issues such as data privacy, the need for robust and diverse datasets, and the integration of these technologies into existing healthcare systems must be addressed. Nonetheless, the potential rewards are significant, and efforts are underway to overcome these hurdles.
In conclusion, the future of nutrition is being reshaped by machine learning, offering a vision of personalized health and wellness that was once the realm of science fiction. As these technologies continue to evolve and mature, the dream of truly customized supplement doses tailored to the unique needs of each individual is becoming a reality. This revolution in nutrition science not only promises to enhance the efficacy of dietary supplements but also represents a significant step forward in our quest for optimal health.
Tailored Wellness: The Role of Machine Learning in Crafting Individualized Supplement Plans
In the realm of wellness and health optimization, the one-size-fits-all approach is rapidly becoming a relic of the past. The burgeoning field of personalized medicine, particularly in the domain of nutritional supplements, is a testament to the growing demand for bespoke health solutions. At the forefront of this revolution is the application of machine learning models, which are playing a pivotal role in customizing supplement doses to meet the unique needs of individuals. This article delves into how these advanced algorithms are transforming the landscape of tailored wellness, ushering in an era of individualized supplement plans that promise enhanced efficacy and safety.
The genesis of this transformation lies in the recognition of the vast inter-individual variability in response to dietary supplements. Factors such as genetics, age, sex, lifestyle, and even gut microbiota composition can influence how a person metabolizes and benefits from various nutrients. Traditional methods of determining supplement doses, often based on broad population averages, fail to account for this diversity, leading to suboptimal outcomes for many individuals. Enter machine learning, a branch of artificial intelligence that thrives on complexity and variability. By analyzing large datasets encompassing genetic information, health records, lifestyle factors, and responses to different supplement regimens, machine learning models can identify patterns and predict the most effective dosages for each individual.
The process begins with the collection of comprehensive data from individuals, including detailed health assessments, dietary habits, and genetic testing results. This data is then fed into sophisticated machine learning algorithms, which analyze it in the context of existing scientific research on nutrition and metabolism. The algorithms are trained to discern intricate relationships between various factors and the effects of specific nutrients on health outcomes. Over time, as more data is collected and analyzed, these models become increasingly accurate in predicting the optimal supplement doses for individuals, taking into account their unique physiological and lifestyle characteristics.
One of the most compelling aspects of using machine learning for customizing supplement doses is its dynamic nature. Unlike static recommendations, these models can adapt to changes in an individual’s health status, lifestyle, or goals. For instance, if a person undergoes significant weight loss or starts a new exercise regimen, the model can adjust their supplement plan accordingly, ensuring that it remains aligned with their current needs and objectives. This adaptability is crucial for maintaining the relevance and effectiveness of personalized supplement plans over time.
Moreover, the application of machine learning in this context holds the promise of not only optimizing health outcomes but also enhancing safety. By precisely calibrating supplement doses, the risk of adverse effects associated with overconsumption of certain nutrients can be minimized. This is particularly important given the growing trend of self-prescribing supplements, which can lead to dangerous imbalances and interactions.
In conclusion, the integration of machine learning models into the realm of nutritional supplements represents a significant leap forward in the pursuit of tailored wellness. By enabling the customization of supplement doses based on individual characteristics and needs, these advanced algorithms are paving the way for more effective, safe, and personalized health interventions. As research in this area continues to evolve, we can anticipate a future where personalized supplement plans, guided by machine learning, become a cornerstone of preventive health care and optimal well-being.