Machine Learning Models for Customizing Supplement Doses
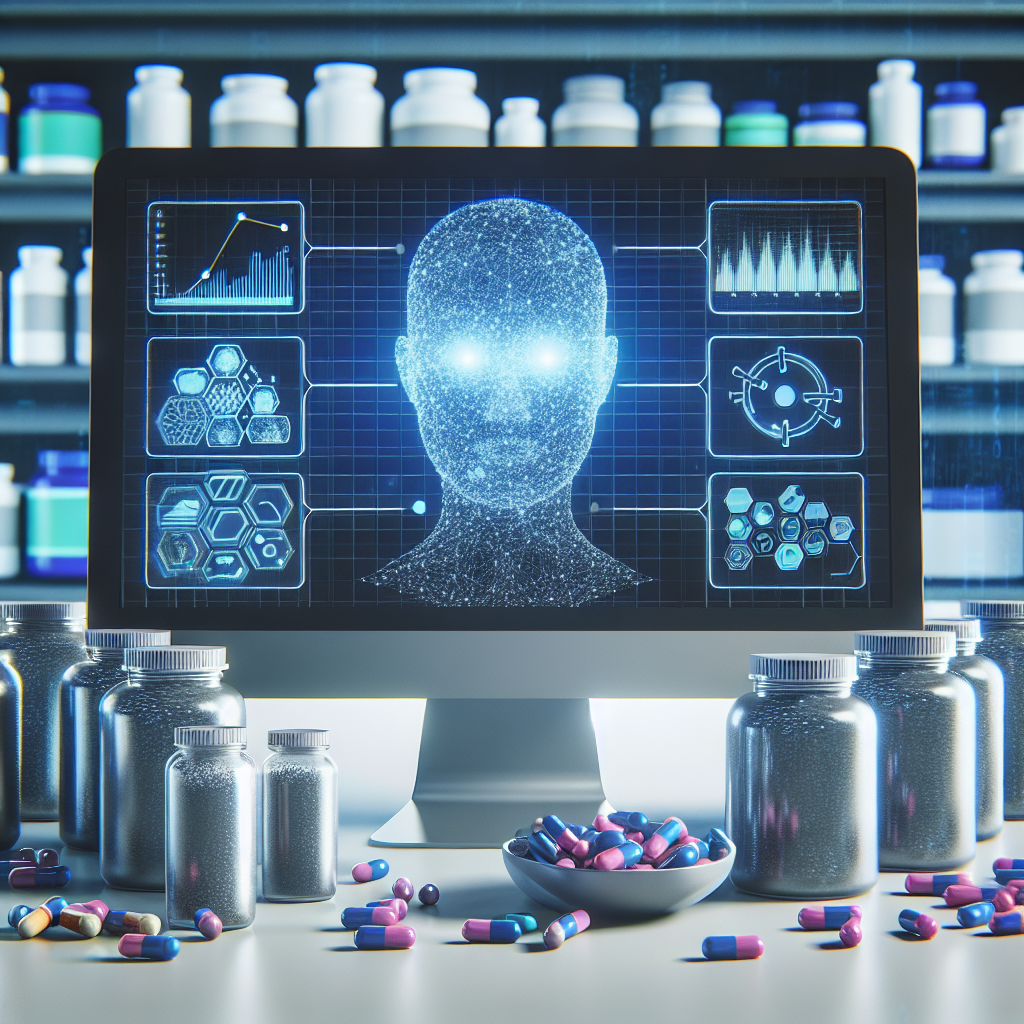
Unlock the full potential of your health with our cutting-edge Machine Learning Models, designed to customize supplement doses tailored specifically for you. Take the first step towards a more vibrant and personalized wellness journey. Discover more and get started today!
Optimizing Health: Leveraging Machine Learning Models for Personalized Supplement Dosages
In the realm of health and wellness, the adage “one size fits all” is increasingly becoming a relic of the past. The advent of machine learning models in healthcare is ushering in an era of personalized medicine, where treatments and interventions can be tailored to the individual’s unique genetic makeup, lifestyle, and health status. Among the most promising applications of this technology is the customization of supplement doses to optimize health outcomes. This approach not only promises to enhance the efficacy of supplements but also to minimize the risk of adverse effects associated with improper dosing.
The journey towards personalized supplement dosages begins with the collection and analysis of vast amounts of data. Machine learning models, with their ability to sift through and make sense of complex datasets, are at the forefront of this endeavor. These models can analyze data from a variety of sources, including genetic tests, blood work, dietary habits, and even activity levels, to identify patterns and correlations that might not be apparent to human researchers. By integrating this information, machine learning algorithms can predict the most effective supplement dosage for an individual, taking into account factors such as metabolism, nutrient absorption rates, and potential interactions with other medications or supplements.
One of the key benefits of using machine learning models for this purpose is their ability to continuously learn and adapt. As more data becomes available, either from the broader population or from an individual’s changing health status and lifestyle, the models can update their predictions to reflect this new information. This dynamic approach ensures that supplement dosages remain optimized over time, even as an individual’s health needs evolve.
Moreover, machine learning models can help to identify the synergistic effects of different supplements, enabling the creation of personalized supplement regimens that maximize health benefits. For instance, certain vitamins and minerals may be more effective when taken together, or in specific ratios, and machine learning can help to uncover these complex interactions. This level of customization is beyond the reach of traditional approaches to supplement dosing, which often rely on standardized guidelines that do not account for individual variability.
However, the implementation of machine learning models in customizing supplement doses is not without challenges. Ensuring the privacy and security of personal health data is paramount, as is the need for rigorous validation of the models to prevent errors that could lead to harmful recommendations. Additionally, there is the question of accessibility, as the benefits of personalized supplement dosages should be available to all, not just those who can afford the latest technology.
Despite these challenges, the potential of machine learning models to revolutionize the way we approach supplement dosing is undeniable. By enabling a more personalized approach to health and wellness, these models can help individuals achieve optimal health outcomes while minimizing the risk of adverse effects. As research in this area continues to advance, we can expect to see an increasing integration of machine learning models into health care practices, marking a significant step forward in the journey towards truly personalized medicine.
Revolutionizing Nutrition: The Role of AI in Tailoring Supplement Intakes
Machine Learning Models for Customizing Supplement Doses
In the realm of nutrition and health, the one-size-fits-all approach is rapidly becoming a relic of the past. The advent of artificial intelligence (AI) and machine learning (ML) technologies has ushered in a new era where personalized nutrition plans, tailored to an individual’s unique physiological makeup, are not just a possibility but a reality. Among the most promising applications of these technologies is the customization of supplement doses, a development that promises to revolutionize the way we think about and consume dietary supplements.
Traditionally, the recommended dosages for vitamins, minerals, and other supplements have been based on broad population averages. However, these recommendations often fail to account for the vast differences in individual needs, influenced by factors such as age, gender, health status, lifestyle, and even genetic predispositions. This is where machine learning models come into play, offering a sophisticated solution to the challenge of personalizing supplement intakes.
Machine learning, a subset of AI, involves the development of algorithms that can learn from and make predictions or decisions based on data. In the context of nutrition, these models can analyze a wide array of information about an individual, including dietary habits, physical activity levels, blood test results, and genetic markers, to predict the optimal dosages of various supplements for that person. This approach not only promises to enhance the effectiveness of supplements but also to minimize the risk of adverse effects associated with overconsumption.
The process begins with the collection of detailed personal health data, which is then fed into the machine learning model. Through a method known as predictive analytics, the model assesses this data to identify patterns and correlations that may not be immediately apparent to human nutritionists or dietitians. For instance, it might uncover that individuals with a certain genetic variant respond better to higher doses of Vitamin D compared to the general population. Armed with these insights, the model can then recommend a customized supplement regimen that is precisely tailored to the individual’s unique needs.
Moreover, the flexibility and adaptability of machine learning models mean that they can continuously refine their recommendations based on new data. As individuals provide feedback on their health outcomes or as new health data becomes available, the models can adjust their predictions to ensure that the supplement doses remain optimized over time. This dynamic approach stands in stark contrast to the static nature of traditional dietary guidelines and represents a significant leap forward in our ability to provide truly personalized nutrition advice.
The integration of machine learning models into the field of nutrition is not without its challenges. Concerns regarding data privacy, the need for large and diverse datasets to train the models, and the importance of ensuring that the recommendations are interpretable and actionable for both individuals and healthcare providers are among the hurdles that must be overcome. However, the potential benefits of this technology, in terms of improving health outcomes and personalizing healthcare, are immense.
In conclusion, the application of machine learning models to customize supplement doses is at the forefront of the revolution in personalized nutrition. By leveraging the power of AI to analyze complex health data, we are moving closer to a future where dietary supplements can be tailored to meet the specific needs of each individual, enhancing their effectiveness and reducing the risk of adverse effects. This represents a significant step forward in our quest for optimal health and well-being, demonstrating the transformative potential of technology in the field of nutrition.
Beyond One-Size-Fits-All: How Machine Learning Transforms Supplement Dosing for Individual Needs
In the realm of health and wellness, the adage “one size fits all” is rapidly becoming a relic of the past, especially when it comes to dietary supplements. The burgeoning field of machine learning is at the forefront of this transformation, offering personalized solutions that promise to revolutionize how individuals approach their supplement intake. This article delves into the innovative application of machine learning models in customizing supplement doses, ensuring that individuals receive the optimal benefits tailored to their unique physiological needs.
The journey into personalized supplement dosing begins with an understanding of the inherent variability in human biology. Factors such as age, gender, genetic predispositions, lifestyle choices, and even the microbiome composition play a crucial role in determining how an individual’s body absorbs and utilizes nutrients. Traditional approaches to supplement dosing have largely ignored this complexity, offering standardized recommendations that may not suit everyone. However, machine learning models are adept at handling vast amounts of data, including these biological variables, to predict more accurate supplement dosing that aligns with individual health goals and needs.
Machine learning, a subset of artificial intelligence, employs algorithms that can learn from and make predictions or decisions based on data. In the context of supplement dosing, these models are trained on datasets that include information on individual responses to various supplement types and doses, health outcomes, and even genetic markers. Over time, as more data is collected and fed into these models, their predictive accuracy improves, enabling highly personalized supplement recommendations.
One of the most compelling aspects of using machine learning for supplement dosing is its dynamic nature. Unlike static dosing guidelines, machine learning models can continuously update and refine dosing recommendations as they receive new data. This means that an individual’s supplement plan can evolve in response to changes in their health status, lifestyle, or even advancements in nutritional science. This adaptive approach ensures that supplement regimens remain optimally beneficial over time, catering to the body’s changing needs.
Moreover, machine learning models can identify patterns and correlations that may not be immediately apparent to human researchers, uncovering novel insights into how different factors influence nutrient needs. For instance, these models might reveal that individuals with a specific genetic marker require higher doses of a particular vitamin to achieve the same health outcomes as those without the marker. Such insights can lead to more effective and personalized supplement strategies that are grounded in scientific evidence.
However, the implementation of machine learning in supplement dosing is not without challenges. Ensuring the privacy and security of personal health data is paramount, as is the need for robust, high-quality datasets to train the models. Additionally, there is a need for collaboration between technologists, nutritionists, and healthcare providers to translate machine learning insights into practical dosing recommendations that can be easily integrated into individuals’ health routines.
In conclusion, machine learning models are setting the stage for a new era in dietary supplement use, moving beyond the one-size-fits-all paradigm to offer personalized dosing recommendations. By harnessing the power of data and advanced analytics, these models promise to enhance the efficacy of supplements, helping individuals achieve their health and wellness goals with unprecedented precision. As research and technology continue to advance, the potential for further personalization and improved health outcomes through tailored supplement dosing appears boundless, marking a significant leap forward in personalized nutrition and healthcare.