Predictive AI Tools for Identifying Nutrient Deficiencies Early
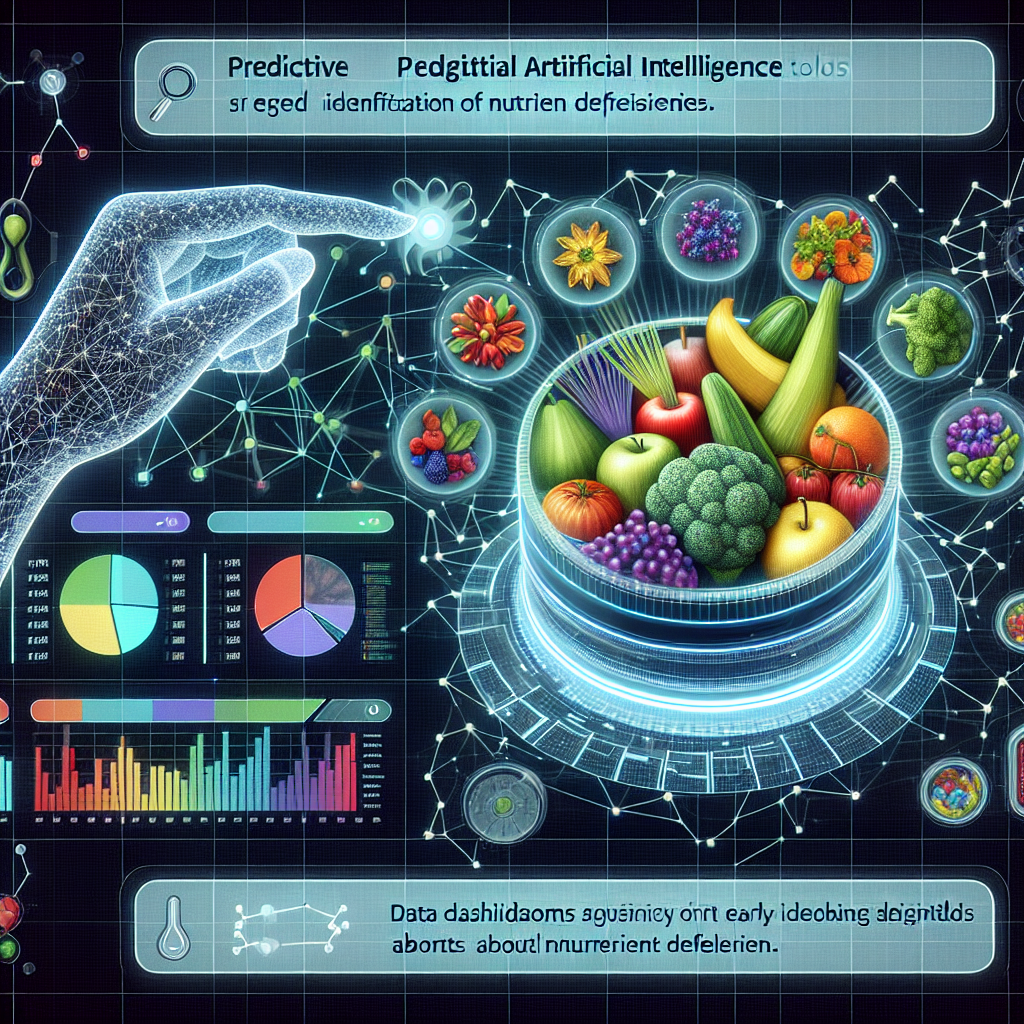
Leveraging Predictive AI Tools for Early Detection of Plant Nutrient Deficiencies
In the realm of agriculture, ensuring the health and productivity of crops is paramount. One of the critical challenges that farmers and agronomists face is the early detection of nutrient deficiencies in plants. These deficiencies can significantly impact crop yield, quality, and overall farm profitability. However, with the advent of predictive AI tools, there is a promising horizon for identifying these deficiencies well before they manifest visibly, allowing for timely intervention and remediation.
Predictive AI tools leverage a combination of advanced algorithms, machine learning, and vast datasets to analyze and predict potential nutrient deficiencies in crops. These tools are designed to process and interpret complex data from various sources, including soil samples, weather patterns, and historical crop performance data. By integrating this information, AI systems can identify subtle signs of nutrient stress in plants that are not immediately apparent to the human eye.
One of the most significant advantages of using predictive AI in agriculture is its ability to provide precise and personalized recommendations for nutrient management. Unlike traditional methods, which often rely on generalized guidelines, AI can tailor its recommendations based on the specific conditions of each farm. This precision agriculture approach ensures that crops receive exactly what they need, when they need it, minimizing waste and optimizing resource use.
Moreover, predictive AI tools can continuously monitor crop health and soil conditions in real-time. This ongoing surveillance enables the early detection of nutrient deficiencies, often before they can cause irreversible damage to the plants. By receiving early warnings, farmers can take proactive measures, such as adjusting fertilization strategies or modifying irrigation practices, to address the identified deficiencies and prevent yield loss.
The implementation of predictive AI in agriculture also holds the potential to revolutionize how farmers approach crop management. By relying on data-driven insights, farmers can make more informed decisions, reducing the reliance on trial and error and the overuse of fertilizers and other inputs. This not only enhances the sustainability of farming practices but also contributes to environmental conservation by reducing runoff and the potential for nutrient pollution in water bodies.
Furthermore, predictive AI tools are becoming increasingly accessible to farmers worldwide, thanks to advancements in technology and the proliferation of mobile devices. With user-friendly interfaces and cloud-based platforms, these tools can deliver actionable insights directly to farmers’ smartphones or computers, making it easier than ever to implement precision agriculture techniques.
In conclusion, the integration of predictive AI tools in agriculture offers a transformative approach to managing plant nutrient deficiencies. By enabling the early detection and precise correction of these deficiencies, AI technologies can help ensure optimal crop health and yield. As these tools continue to evolve and become more widely adopted, they promise to play a crucial role in advancing sustainable agriculture practices and securing the global food supply. The future of farming lies in harnessing the power of AI to anticipate challenges, optimize resources, and sustainably produce the food that feeds the world.
The Role of Predictive AI in Identifying Human Nutritional Deficiencies Before They Manifest
Predictive AI Tools for Identifying Nutrient Deficiencies Early
In the realm of healthcare and nutrition, the adage “prevention is better than cure” holds profound significance. With the advent of advanced technologies, particularly artificial intelligence (AI), the ability to foresee and mitigate health issues before they escalate has remarkably improved. Among these advancements, predictive AI tools have emerged as a groundbreaking approach in identifying human nutritional deficiencies early, potentially revolutionizing how we address and manage health and wellness.
Predictive AI, with its capacity to analyze vast datasets and identify patterns that are imperceptible to the human eye, is at the forefront of this innovation. These tools leverage machine learning algorithms to sift through and interpret complex nutritional data, drawing correlations between dietary habits, health outcomes, and the risk of nutrient deficiencies. This capability not only enhances our understanding of nutrition but also enables personalized dietary recommendations, tailored to the unique needs of individuals.
The significance of early detection of nutrient deficiencies cannot be overstated. Nutritional deficiencies, if left unchecked, can lead to a myriad of health issues, ranging from fatigue and weakened immunity to severe chronic diseases. Traditional methods of identifying these deficiencies often rely on the manifestation of physical symptoms or the onset of related health conditions. However, by this stage, rectifying the deficiency can be more challenging, necessitating more intensive interventions. Predictive AI tools, by contrast, offer a proactive solution, identifying risks before they manifest into more serious health problems.
Moreover, these AI-driven tools are not just limited to predicting potential deficiencies; they also play a crucial role in formulating preventive strategies. By analyzing individual dietary patterns and lifestyle factors, predictive AI can recommend adjustments to one’s diet to avert the risk of developing deficiencies. This personalized approach to nutrition underscores the shift towards more individualized healthcare, where interventions are tailored to the specific needs and risks of each person.
The integration of predictive AI in identifying nutritional deficiencies also holds promise for public health initiatives. By aggregating and analyzing population-level data, these tools can identify at-risk groups, guiding targeted nutritional interventions and policies. This could be particularly beneficial in addressing nutritional deficiencies in underserved communities, where access to healthcare and nutritional education may be limited.
However, the implementation of predictive AI in nutrition and healthcare is not without challenges. Concerns regarding data privacy, the need for robust datasets to train AI models, and the importance of ensuring these tools are accessible to all segments of the population are critical considerations. Moreover, the reliance on AI-driven recommendations necessitates a level of trust in these technologies, underscoring the need for transparency and validation of these tools through rigorous scientific scrutiny.
In conclusion, predictive AI tools represent a significant leap forward in our ability to identify and prevent nutritional deficiencies before they manifest. By harnessing the power of AI to analyze complex datasets and predict potential health risks, we can move towards a more proactive and personalized approach to nutrition and healthcare. As these technologies continue to evolve, the potential for improving health outcomes and enhancing the quality of life for individuals around the globe is immense. The journey towards integrating predictive AI into our healthcare systems is just beginning, but the promise it holds is a testament to the transformative power of technology in shaping a healthier future.
Advancements in Predictive AI for Precision Agriculture: Tackling Nutrient Deficiency Early On
In the realm of precision agriculture, the advent of predictive AI tools has marked a significant leap forward, particularly in the early identification of nutrient deficiencies in crops. This innovative approach not only promises to revolutionize the way farmers manage crop nutrition but also paves the way for more sustainable agricultural practices. By leveraging the power of artificial intelligence, these tools are set to transform the agricultural landscape, ensuring that crops receive the precise nutrients they need, exactly when they need them.
The journey towards the development of predictive AI tools for identifying nutrient deficiencies early on begins with the understanding that timely and accurate diagnosis is crucial for optimal crop health and yield. Traditionally, farmers have relied on physical symptoms to detect nutrient deficiencies, a method that often leads to delayed intervention and, consequently, reduced crop productivity. However, with the integration of AI into precision agriculture, this reactive approach is swiftly being replaced by a proactive strategy that identifies potential issues before they visibly affect plant health.
Predictive AI tools employ a variety of techniques, including machine learning algorithms and data analytics, to analyze vast amounts of data from different sources. These sources range from satellite imagery and drone photos to soil and weather data, all of which contribute to a comprehensive understanding of the crop’s environment. By processing this data, AI algorithms can detect subtle changes in plant health that are indicative of nutrient deficiencies, often long before these deficiencies manifest as visible symptoms. This early detection is crucial for implementing corrective measures in a timely manner, thereby minimizing the impact on crop yield.
Moreover, these AI-driven tools are not only capable of identifying existing nutrient deficiencies but also predicting future risks. This predictive capability is grounded in the analysis of historical data and current conditions, allowing for the anticipation of nutrient imbalances before they occur. Consequently, farmers can adjust their fertilization strategies based on AI recommendations, optimizing nutrient management and reducing the risk of over- or under-fertilization. This precision in nutrient application not only enhances crop health and yield but also contributes to environmental sustainability by minimizing the runoff of excess nutrients.
The implementation of predictive AI tools in agriculture also brings about a significant shift in resource allocation. By pinpointing exactly where and when nutrients are needed, these tools enable farmers to allocate their resources more efficiently, reducing waste and lowering costs. This targeted approach to nutrient management is particularly beneficial in large-scale farming operations, where the optimization of resources can lead to substantial economic gains.
In conclusion, the integration of predictive AI tools into precision agriculture represents a transformative development in the early identification and management of nutrient deficiencies. By harnessing the power of artificial intelligence, these tools offer a proactive solution that enhances crop health, maximizes yield, and promotes environmental sustainability. As the agricultural sector continues to evolve, the adoption of AI-driven technologies such as these will undoubtedly play a pivotal role in shaping the future of farming, ensuring that it is both productive and sustainable.